What is Coronal Mass Ejection?
A coronal mass ejection (CME) is a significant release of plasma and accompanying magnetic field from the Sun’s corona into the heliosphere. CMEs are often associated with solar flares and other forms of solar activity, but a broadly accepted theoretical understanding of these relationships has not been established.
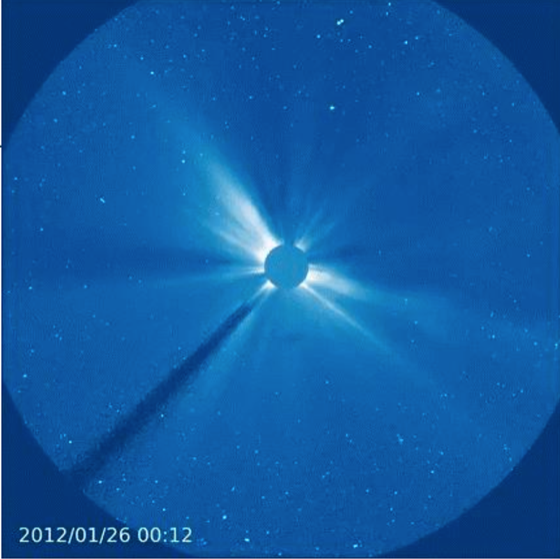
Image: Coronal Mass Ejection Detection captured by SOHO in 2012. Image Courtesy of ESA
2.Using AI for Coronal Mass Ejection detection
In the context of Space Situational Awareness (SSA), on-board detection of space weather events – such as solar flares or Coronal Mass Ejections (CME) – can decrease the system latency for critical information reaching the end-users. Unfortunately, such detection requires complex on-board image analysis not commonly deployed on institutional missions, due to lack of high-performance space qualified processors and available qualified software tools. However, in recent years there has been an increasing use of on-board machine learning inference in NewSpace missions – such as the achievements of the inclusion of an AI-accelerator processor on-board Phisat-1, allowing an increase in on-board autonomy.
ESA has developed and optimised two machine learning applications based on ANN (Artificial Neural Networks) targeting Space Weather applications: on-board Coronal Mass Ejection detection and detection of radiation upsets in optical imagers for on-board radiation scrubbing. The Coronal Mass Ejection detection algorithm was initially trained and provided by University di Torino, which was later retrained at ESTEC and adapted for candidate hardware targets.
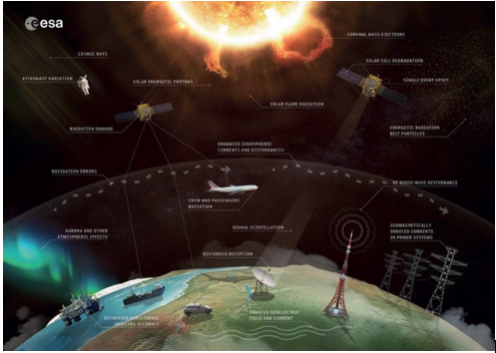
Image: Space Weather On-Board Detection. Image Courtesy of ESA
3.KATESU project
The current commercial version of Klepsydra AI has been successfully validated in an ESA activity called KATESU for Teledyne e2v’s LS1046 and Xilinx ZedBoard onboard computers with outstanding performance results. As part of this activity two DNN algorithms which form part of the provided by ESA were tested: CME and OBPMark-ML benchmarking suite were provided, cloud screening and Coronal Mass Ejection Detection.
How to run the demo
Step 1
The following link has to be used to start the DEMO:
There are two versions of the demo:
- For x86: https://space.demo.klepsydra.com/esa-admin/
- For ARM: https://spacearm.demo.klepsydra.com/esa-admin/
Firstly, go to the link and wait 5 seconds for the table to show the status of the demo (active or inactive). If they are in green, the demo is running correctly. However, if they are red, the demo has stopped or finished (it lasts 20 mins). If the demo is not active (RED), then press the start button and wait 5 seconds for the demo to start:
Step 2
If all four boxes are green, including the text OK, it means the demo was started correctly. After the demo is started and processes are running, press the “go to demo” button:
This button takes you to the demo below:
Step 3
Once selected, a list of boxes will appear on the right. Click on the one named “CME demo”:
4.Benefits of Klepsydra AI
The demo shows three executions of the Coronal Mass Ejection Detection DNN model in three identical computers. The first one running Klepsydra optimised for latency (labelled as kpsr.lat), the second is executed using TenserFlowLite and the third one using Klepsydra optimised for CPU (labelled as kpsr.cpu).
This demo shows the elasticity and high performance results of Klepsydra AI: kpsr.lat can process up to 2 times more images per second with respect to TensorFlow Lite, while kpsr.cpu processes the same amount of images as per TensorFlowLite but with less CPU. This performance improvement can be seen in both Intel and ARM variations of the demo.
In summary, Klepsydra AI provides users with the unique feature of being able to adapt to the customer requirements, maybe that be latency, CPU, RAM or throughput. This feature makes Klepsydra AI very well suited for onboard AI applications for Earth Observation onboard data processing and compression, Vision-based navigation for in-orbit servicing and Lunar landing.
Acknowledgments
This demo was prepared as part of ESA’s KATESU project to evaluate Klepsydra AI for Space use. For further information on this project, please refer to https://klepsydra.com/klepsydra-ai-technology-evaluation-space-use/.
The Coronal Mass Ejection Detection DNN was provided to Klepsydra by courtesy of ESA. This algorithm is part of ESA’s OBPMark framework. For further information on this framework, please contact OBPMark@esa.int.